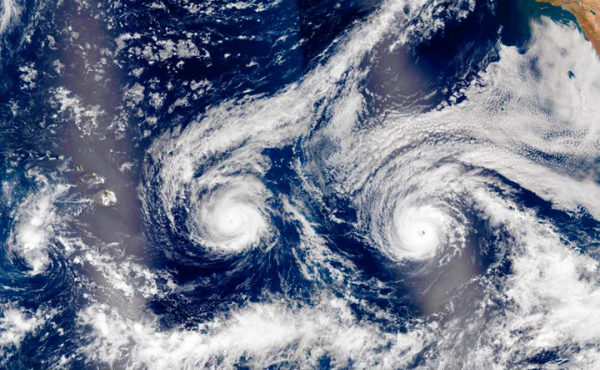
气象学一直都在努力解决大数据的问题。天气具有多变性和混沌性,半个多世纪以来,气象学家一直在试着处理兆兆字节(TB)级别的数据和建模变量做准确的天气预测。到了今天,我们还是在处理数据,数据规模到了千兆兆字节(PB)级别,拜物联网、更多的传感器和集合建模所赐。(图:Getty上的环球历史档案馆/环球图片集团)
业界已经不乏关于人工智能及其应用的好处的讨论,包括约会、营销和社交媒体到太空探索和医学进步。每个行业都受到人工智能工具的影响,包括气象行业。
Meteorology has always grappled with the problem of big data. I would even propose that the science was the epitome of big data before the word became mainstream. Due to the multivariate and chaotic nature of weather, for more than half of a century meteorologists have dealt with terabytes of data and modeling variables to produce an accurate forecast. Today, we are still processing data – now on the scale of petabytes – thanks to the Internet of Things, more sensors, and ensemble modeling. Writer Ted Alcorn estimates that, “Today’s (weather) models incorporate about 100 million pieces of data each day, a level of complexity comparable to simulations of the human brain or the birth of the universe.”
气象学一直都在努力解决大数据的问题。笔者甚至认为,在大数据这个词成为主流之前,气象学就是大数据的缩影。天气具有多变性和混沌性,半个多世纪以来,气象学家一直在试着处理兆兆字节(TB)级别的数据和建模变量做准确的天气预测。到了今天,我们还是在处理数据,数据规模到了千兆兆字节(PB)级别,拜物联网、更多的传感器和集合建模所赐。写手Ted Alcorn估计,“今天的(天气)模型每天用到约1亿条数据,其复杂程度堪比对人脑或宇宙诞生的模拟。”
But computing power and the advancement of technology such as AI have allowed us to not only analyze the data quicker and easier, but also “learn” from historical data for better situational awareness and decision-making. Within the weather community, AI is being applied to several different challenges. One focus is to make a better weather forecast.
但计算能力和人工智能等技术在不断进步,我们现在不仅能够更快、更容易地分析数据,而且还能从历史数据中 “学习”,从而获得更好的态势感知和做出更好的决策。人工智能在气象界能够用于应对几个不同的挑战。其中的一个重点是做出更好的天气预报。
Forecasting is increasingly becoming more accurate. Today a five-day forecast has a 90% accuracy, the same as a three-day forecast 25 years ago. Short-term predictions, or now casting in hourly time spans, is more challenging particularly due to micro changes at the surface. Scientists at DeepMind and the University of Exeter have partnered with the U.K. Met Office to build a nowcasting system using AI that would overcome these challenges to make more accurate short-term predictions, including for critical storms and floods. Another research study is looking at the efficiency of modeling and how AI can analyze past weather patterns to predict future events, more efficiently and more accurately.
天气预报现在可以更加准确了。时下五天的预测有90%的准确性,与25年前三天预测的准确度相同。短期预测(也就是说以小时为时间跨度的当下预测)则更具挑战性,主要是由于地面的微观变化。DeepMind和埃克塞特大学的科学家们与英国气象局合作,利用人工智能建立了一个当下预测系统,该系统可望克服这些挑战做出更准确的短期预测,包括对重大风暴和洪水的预测。另外一项研究是研究建模的效率以及人工智能如何分析过去的天气模式对未来事件更有效和更准确地预测。
My focus of work – and the area of AI that I am particularly interested in – is its application to predict the potential impact from weather events. The outcomes of weather as opposed to the weather itself.
笔者的工作重点(以及我特别感兴趣的人工智能领域)是人工智能在预测来自天气事件的潜在影响方面的应用,相对于天气本身而言,更多的涉及到天气所产生的结果。
For example, using AI in the utility sector to predict potential outages. Historical outage data is collected on a specific utility location, or region, and allows a computer to generate predictions for future needs based on forecasted weather conditions. It understands how infrastructure has responded to past storms including learning differences in network hardening, realizing the age of individual infrastructure components and maintenance practices. These datasets will yield a baseline of potential outages from upcoming storms. We can apply the same approach with municipalities. Understanding variables such as the city’s infrastructure, topography, and evacuation routes, along with historical weather data, we can help cities have better insight into potential areas of impact and risk of public or infrastructure safety.
例如,公共事业部门利用人工智能预测可能出现的停电。一个特定的公用事业地点或地区的历史停电数据收集了以后可以允许计算机根据预测的天气状况生成对未来需求的预测。这些历史数据涵括了基础设施应对过去的风暴的知识,包括学习加固网络的差异,实现个别基础设施组件的年龄和维护的做法。这些数据集可以生成未来可能到来的风暴导致的停电基线数据。市政区管理层次上也可以采用同样的方法。我们对于城市的基础设施、地形和疏散路线等变量以及历史气象数据的了解,可以帮助城市更好地洞察潜在的影响领域和公共或基础设施安全的风险。
And, while we talk about advanced technology and insights, I think it is important to note that the human element is still crucial to the process. A recent Wired article citied studies that found forecasts by human forecasters were more accurate than AI forecasts.
而且,在我们谈论先进的技术和洞察力的同时,笔者认为人的因素在这个过程中仍然至关重要。最近《连线》杂志的一篇文章提到一些研究结果,这些研究发现人类预报员的预测比人工智能的预测更准确。
Another area that requires human intervention is the increasing need for risk communicators. These are meteorologists who take the forecast further and convey the risk or impact to a business, municipality or public. I have heard several comments that when AI is more trustworthy it will be as simple as toggling weather preferences to have accurate, meaningful weather data on demand. While I agree that we will have progressively better data and forecasts, I believe this will also increase the need for human experts to evaluate, interpret and communicate the data – and the risk and impact – in a way that makes sense to those who must make nimble, informed decisions to protect people, infrastructure, and businesses assets. The bigger question shouldn’t be human or AI forecasts, but rather how can meteorologists use improved AI to help decision makers make the best decisions for their stakeholders.
另一个需要人为干预的领域是风险沟通者的增长需求。风险沟通者是一群特殊的气象学家,他们在预测的基础上更进了一步,将风险或影响传达给企业、市政当局或公众。我听到过一些评论指,在人工智能更值得信赖后就可以简单地切换天气偏好按需求获得准确、有意义的天气数据。我们将拥有逐步改善的数据和预测,这一点我同意,但我相信这也将增加对人类专家的需求,以达到评估、解释和沟通数据(以及风险和影响)的目的,所用到的方式是有意义的,可以帮助那些必须做出灵活、明智决定以保护人民、基础设施和企业资产的人。更大的问题并不是人类预测还是人工智能预测,而是气象学家如何利用改进的人工智能帮助决策者为他们的利益相关者做出最佳决定。